IROS 2023 CLiFF-LHMP: Using Spatial Dynamics Patterns for Long-Term Human Motion Prediction
Community
IROS 2023: Predicting Human Movement
NEWS, Community, Robotics, Perception, Artificial Intelligence |
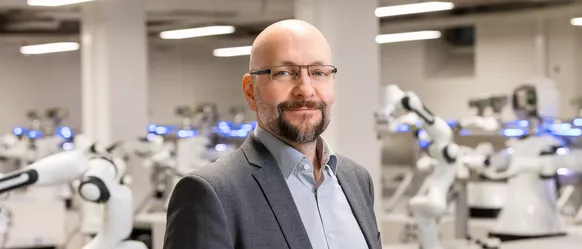
The idea was promising: Spencer greets passengers at the entrance of Amsterdam's Schiphol Airport, checks their boarding passes, and escorts them to the gate. While this might be relatively straightforward in an empty hall, it becomes a challenge in a crowded space. Spencer's goal is to reach the gate as quickly as possible while minimizing disruption to people's movements. Finding the best path to the destination was not easy because, based on the path planning that was state-of-the-art in 2016, the mobile robot often chose a path that went against the predominant direction of human movement. This happened because, at that time, robots could only incorporate movements perceived in their immediate surroundings into their path planning, without considering typical movement patterns prevailing in an environment. Instead of guiding passengers to their gate, the robot would often get stuck in a crowd of people, hindering their progress.
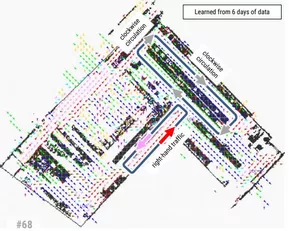
92 Days of Data from a Shopping Center in Japan
Professor Achim Lilienthal, who specializes in the perception of intelligent systems at the Technical University of Munich (TUM), was instrumental in the development of Spencer. Inspired by their experiences at Amsterdam's airport, he and colleagues established the research field known as 'Maps of Dynamics.' This involves techniques that allow robots to create maps from directly observed movement, recording prevailing movement patterns in an environment. With the additional information in these maps (such as the likelihood that people at a specific point will slowly turn left or continue straight ahead quickly), robots can now navigate through crowds more effectively.
More Precise Predictions: Deviations of Only Five Meters after 50 Seconds
Initially focused on improving robot planning capabilities, the work presented at this year's IROS aims to make more accurate predictions about the future movements of individual people who are briefly near the robot, using 'Maps of Dynamics.' For this research, the MIRMI professor and his team used 92 days of spatial movement data recorded in a Japanese shopping center. Historical consumer movement data indicate the most common directions people tend to move. With this data, researchers can predict where people will move much more reliably than before. When comparing methods, under Lilienthal's direction at the Technical University of Munich (TUM), after 50 seconds, the average deviation from the stated target is only five meters, compared to eight to 12 meters with previous state-of-the-art approaches. This represents a breakthrough in predicting movements over such extended time periods.
The Goal: Autonomous Mobile Robots Should Be Socially Compatible
If these findings had been applied to Spencer, the robot at Amsterdam's airport would not have gone against the flow and could have predicted the movements of individual people over longer periods more accurately. These methods are crucial for the safety and acceptance of autonomous mobile robots. When a person or vehicle approaches, the robot often has only two options: stop or jeopardize the safety of the surroundings. With knowledge accessible through Maps of Dynamics about how people move even beyond the directly 'observable' area, the risk of collisions can be minimized, and the risk of getting stuck in traffic or against the flow can be reduced. In this way, autonomous mobile robots can be integrated into environments shared with humans more safely and socially acceptably.
Paper: CLiFF-LHMP: Using Spatial Dynamics Patterns for Long-Term Human Motion Prediction; Yufei Zhu, Andrey Rudenko, Tomasz P. Kucner, Luigi Palmieri, Kai O. Arras, Achim J. Lilienthal, Martin Magnusson; IROS 2023